Turkey Becomes ‘Country of Suspects’
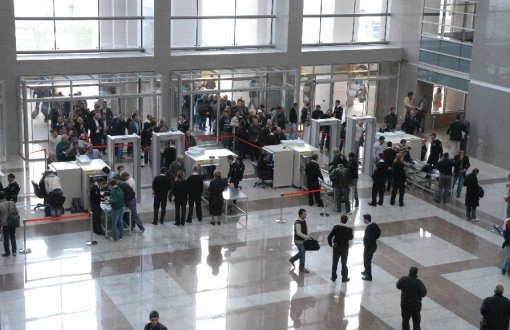
Click to read the article in Turkish
The number of investigations, prosecutions and legal proceedings surrounding Turkey have come to an unsolvable stage already in the wake of July 15 coup attempt.
In addition to governing party, the Justice and Development Party (AKP), academics and jurists arrange meetings for alternative solutions.
Does increasing the number of chambers and members solve the issue?
Speaking at the “Alternative Solutions Before Judgement” organized by the Faculty of Law at Okan University on November 23, 2017, President of the Supreme Court İsmail Rüştü said:
“It is obvious that increasing the number of chamber and members at the Supreme Court will not solve the workload problem”.
Meant by the “workload” is completing legal proceedings of the number of suspects amounting 8% of the population.
This data was obtained from criminal record stats in 2016. According to the data, eight out of every 100 people are suspects in the eyes of the state.
Number of lawsuits has increased
The number of lawsuits was 5 million 934 thousand 915. The figure reached 6 million 718 thousand 079 in 2016.
The number of lawsuits per judge has decreased
The number of lawsuits per judge was 1,010 in 2007 and the figure dropped to 725 seeing a decrease by 28.2% in 2016.
The number of lawsuits in penal courts has decreased by 21.3%; has increased by 47% in civil courts and by 64.5% in administrative justice courts over the last 10 years.
FETÖ operations
In its statement on October 22, the Ministry of Justice stated that 49 thousand 697 people have been arrested; detention warrants have been issued for 8 thousand 997; 738 people have been in detention as part of the “Fethullahist Terrorist Organization (FETÖ)” operations. (HK/TK)